Predictive analytics: an in-depth look to improve your business decisions
Predictive Analytics, along with Data Science, delivers corporations detailed information about their data. With this, it becomes possible to create future forecasts and make decisions in a much more strategic way. Follow our article to understand how!
Technology has evolved, and today it is possible to analyze possible scenarios. Futurism, Artificial Intelligence, Data Science, and Machine Learning are all technological pillars that, in the hands of skilled and qualified professionals, can help understand the past and build possibilities for the future.
Predictive analytics is not magic, nor is it predicting the future. Predictive analytics is anticipating possible future scenarios through data. It’s a way to use the past and present to project the future, mapping probabilities based on what has already happened.
Predictive analytics doesn’t answer “what’s going to happen.”
Predictive analytics answers “what is likely to happen.”
If you could analyze your choices before making them, you could:
- Increase your performance
- Make more assertive business decisions
- Increase your competitive potential in an increasingly competitive market
- Solve your company’s current pains in a much simpler way
- Grow your ROI exponentially
Let’s analyze this in parts. First, we’ll explain what predictive analytics is and how it works.
What is predictive analytics?
Predictive analytics is a data analysis technique that uses statistical algorithms and Machine Learning techniques to identify the likelihood of future scenarios from historical data.
In short: predictive analytics is an accurate calculation of probabilities based on processing large volumes of data.
Combining data science and predictive analytics allows corporations to obtain detailed information about their data and create future predictions without guesswork or intuition.
There’s nothing esoteric to it. These are just good data analytics practices to strategically and competitively deal with the growing volume of data.
The purpose of Predictive Analytics is to go beyond knowing what happened, providing a better assessment of what might happen in the future.
For this, it is necessary to fulfill a series of requirements. The key to that is Data Science. Data science is the new black for corporations. Mandatory and necessary.
Why is predictive analytics conquering the market?
Predictive analytics has been around for decades – the concept originated in the 1940s when governments started using the first computers. In other words, it’s not exactly new for corporations.
The model has been gaining more and more visibility. We are witnessing a movement in the market where more and more organizations are adhering to this type of analysis to increase profitability and gain a competitive advantage.
And why is this happening? There are 3 main reasons:
1 – Technology ↑
Increasingly powerful processors and new technologies are available to corporations.
2 – + fast + simple + cheap
It’s a simple, fast, and cheap solution to improve decision-making.
3 – Big Data!
According to Gartner, it is the set of “high-volume, high-speed, and/or high-variety information assets that require innovative and cost-effective ways of processing, enabling enhanced insights, decision-making, and process automation.”
What we have today is more data, more processors, better technology, and less cost of implementation.
However, to take advantage of this, corporations must have robust Data Science and Machine Learning frameworks, in addition to specific tools and specialized professionals.
6 steps to implement predictive analytics
To create a predictive analytics model, you need to follow some basic steps. Depending on your challenges, business objectives, and even the degree of maturity of your corporation, you can adjust some of these steps.
1. Objective definition
The first step is pretty straightforward: have a project with well-defined business objectives. Remember, if you don’t know which way to go, any direction will do.
The first question to ask is: what is the purpose of my analysis?
2. Choosing Analysis Goals
This is a direct offshoot of the first step. Here, you and your team will define your analytics goals in line with your business objectives.
With this, you will already define the predictive model of your analysis.
3. Time for data
Taking a step back, it’s essential at this stage to have a collection tool suitable for this type of analysis. Whether it’s internal, external, from social networks, specialized consultants, surveys, or senses, you are going to need an extensive database.
Remember: it’s the quality of the data that is most important, not necessarily the quantity. Focus on the data you need to fulfill your objective.
4. Cleaning time
Now it’s time to clean up your data. Going straight to data analysis can be quite dangerous and compromise your result with “dirty” data.
Therefore, it is essential to prepare your selected data.
Remove what is unnecessary and put the rest in the proper format to facilitate analysis.
Then, it’s just a matter of defining the variables, sorting the data, and separating it into specific sets. Here, anything goes from Excel to Power BI. The key is to use a tool that minimizes the possibility of errors while providing agility.
5. Finally, the analysis
Done! You can start the analysis process. Here, it is essential to have a statistical team capable of understanding and evaluating the information post-analysis.
6. Predictive Model
Now you will finally create your predictive model with the selected data. It will be a pattern of mathematical and statistical techniques that will process the data collected from the relationships you built, providing quick and easy-to-visualize answers.
- Important: The idea here is to keep monitoring your model to ensure its accuracy and efficiency. This is the only way to keep results reliable and useful for your business.
- Tip: Set up a periodic review in your model to ensure that your data changes don’t affect your results.
Top Business Applications of Predictive Models
With increasing data production, companies are looking to predictive analytics as a simple, fast, and cost-effective way to solve complex problems and discover new opportunities.
We’ve prepared a list of some predictive model applications in different industries.
If you have challenges to resolve and you don’t know if predictive analytics models can help you or not, ask for help! Professionals specializing in data analysis can see solutions that not only solve problems but also increase your corporation’s competitive potential. Not sure where to start, contact one of our Data Science experts!
Check out our list of predictive analytics applications:
1. Fraud Analysis and Detection
Combining various analytical methods can improve pattern detection and prevent criminal behavior. As cybersecurity becomes an ever-increasing concern, high-performance behavioral analytics examines every action on a network in real-time to identify anomalies that can indicate fraud, zero-day vulnerabilities, and advanced persistent threats.
2. Optimization of marketing campaigns
Predictive analytics is used to determine customer responses or purchases and to promote cross-selling opportunities. Predictive models help companies attract and retain customers, as well as expand their reach.
3. Improve operations
Large retail companies face a variety of operational challenges on a daily basis. Many of the companies in this industry use predictive models to forecast inventory and manage resources.
Complex logistics involves several different technologies. Each of these directly affects the experience of internal and external users. In this sense, predictive analytics allows organizations to function more efficiently.
Even with retailers suffering wave after wave of challenges in the post-pandemic world, it is possible for retail companies to increase their operational fluidity. Learn more about how here!
4. Reduce risk
Credit scores are used to assess the likelihood that a consumer’s purchasing patterns are actually theirs, a well-known example of predictive analytics. A credit score is a number generated by a predictive model that incorporates all the data relevant to a person’s creditworthiness. Other risk-related uses include insurance claims and activations.
MJV validated the proposal for a new business model for providing credit from one of the largest financial institutions in the world. Understand better how we solved this challenge here!
And as we always say, you don’t have to go alone. If you know predictive analytics is the right solution for you, have pain points on fraud and risk, or want to improve your marketing campaigns and operations, hit a consultant here.
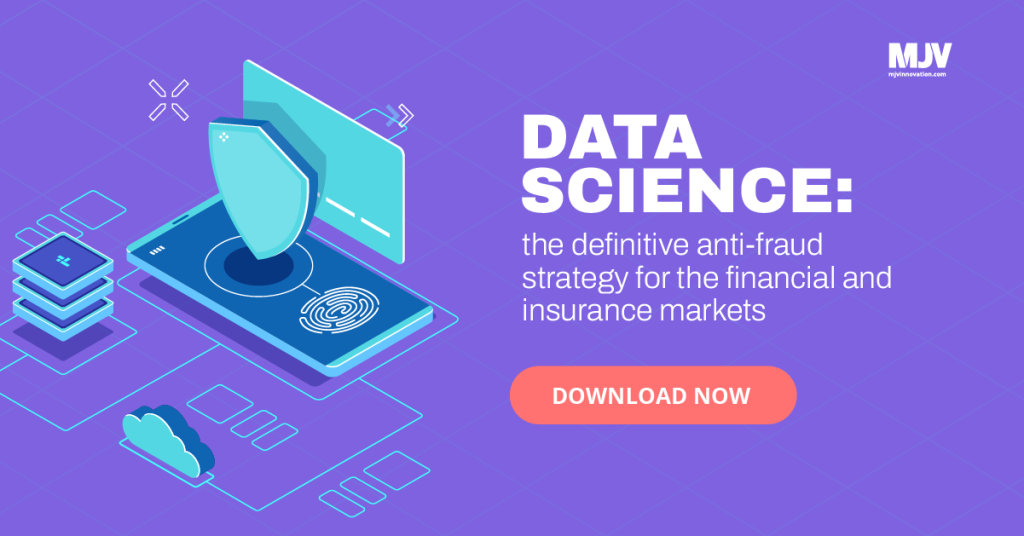